Utilising AI to Enhance Secondary Research Analysis
Background
Secondary research plays a crucial role in providing valuable insights and supporting decision-making processes during our foundation or discovery phases. Our User Centred Design (UCD) team often explores what we refer to as ‘secondary primary’ data, which helps to understand users’ opinions and experiences through existing sources. Examples of this include social media posts, forums, reviews, and blogs. These sources can all provide fantastic insights, but also generate a lot of data and time-consuming analysis.
To address this challenge, the UCD team recently experimented with leveraging Artificial Intelligence (AI) to assist in thematically analysing secondary data. Cat, our Senior User Centred Designer, explains the process they undertook:
“Typically, as researchers we rely on our expertise to manually analyse secondary data sources. However, with multifaceted topics manual analysis can be slow, and tight discovery timelines may result in data being overlooked. This led to us deciding to trial the use of AI during a recent project to accelerate the analysis of secondary data.
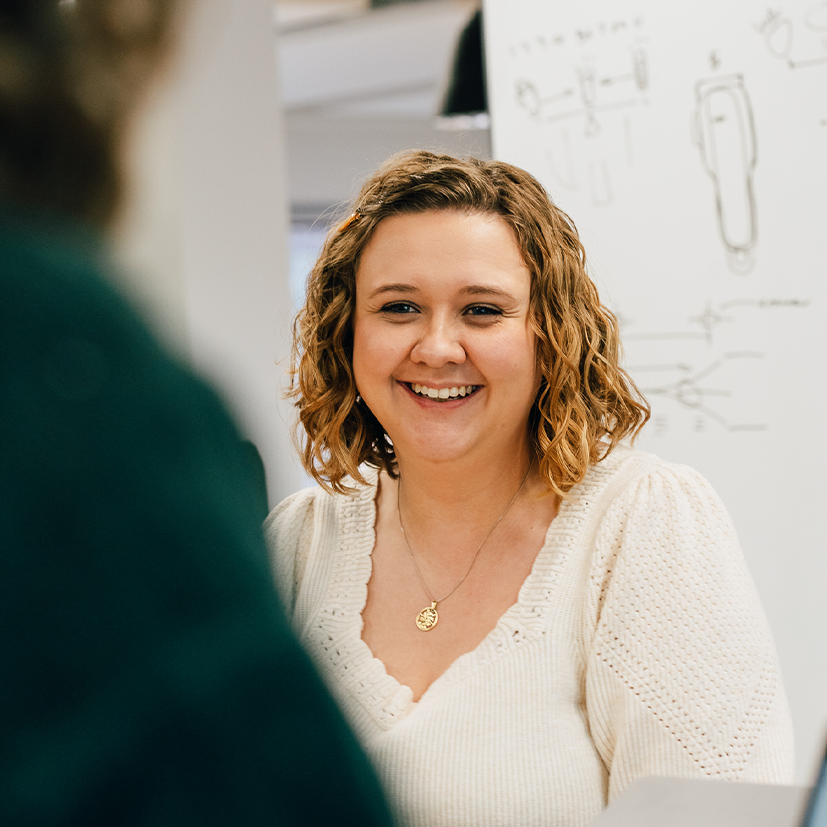
Factors to consider
One initial concern the UCD team had about conducting AI-driven research was the lack of credibility and traceability of the sources being used to inform open AI tools. The team addressed this issue by managing the data that was referenced and only using AI for the analysis stage. This approach allowed the team to maintain control over the data selection process and still immerse themselves in the topic. This facilitated a depth of knowledge and understanding that would have been lost had AI been used to fully substitute the foundation research phase.
Our chosen AI tool to analyse the secondary date for this trial was Miro Assist, part of the Miro collaborative digital whiteboard software that we regularly use at PDR. The AI was asked to thematically analyse the collected data within each research area, as well as produce concise summaries that effectively captured and explained the findings.
Results
The outputs provided valuable insights that guided the subsequent stages of the research process. The themes helped to quickly identify commonalities in the documented experiences of specific user groups, which in turn helped the design of primary research materials such as interview scripts. The final summaries also conveyed the findings in a concise and digestible format that facilitated prompt communication and alignment amongst the wider project team.
Results
The outputs provided valuable insights that guided the subsequent stages of the research process. The themes helped to quickly identify commonalities in the documented experiences of specific user groups, which in turn helped the design of primary research materials such as interview scripts. The final summaries also conveyed the findings in a concise and digestible format that facilitated prompt communication and alignment amongst the wider project team.
Utilising AI tools in this way saved us considerable time while still ensuring dependable and valuable outcomes from secondary research. One of the key advantages of our approach was the ability to combine human expertise with machine capabilities. While AI can efficiently process large volumes of data and identify patterns quickly, it lacks the critical thinking abilities and contextual understanding of human researchers working to variable client and project requirements. By involving the team in the data selection process, we negated any concerns around AI source traceability and enabled more efficient processes and alignment across the wider team.
As technology continues to advance, the interaction between human researchers and AI will undoubtedly play a pivotal role in not only increasing productivity and generating meaningful insights, but also driving innovation and enhancing research practices.”
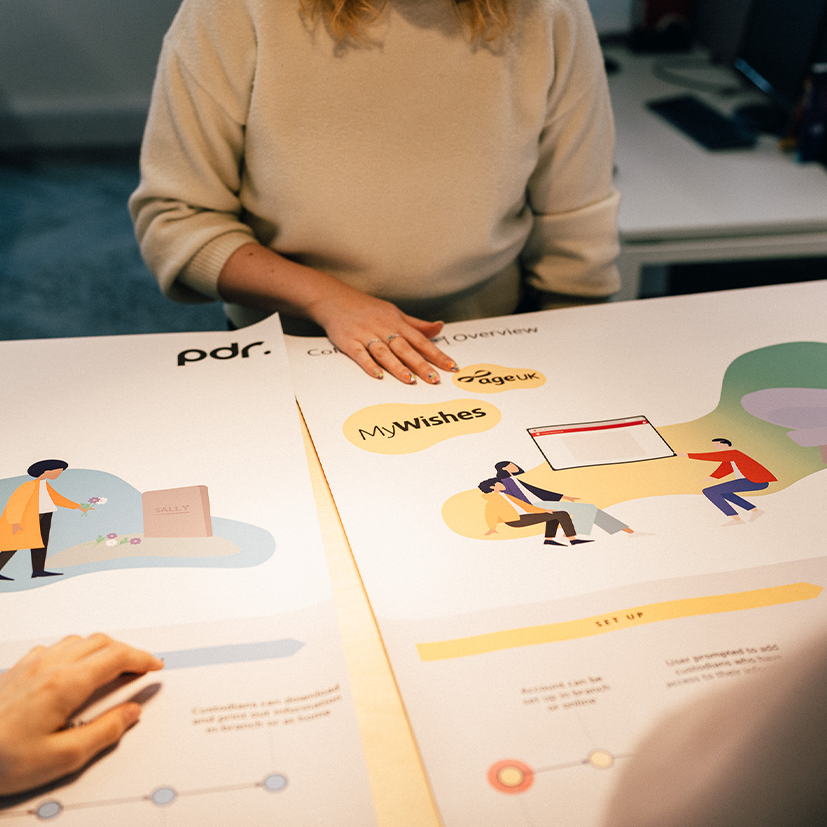